One of the highlights of the upcoming ICT conference is the lineup of esteemed keynote speakers who will be sharing their insights and experiences on the latest trends and developments in the field. These experts are at the forefront of their respective areas, and their presentations will provide attendees with valuable information and inspiration. Among the keynote speakers at the conference, you can expect to hear from..
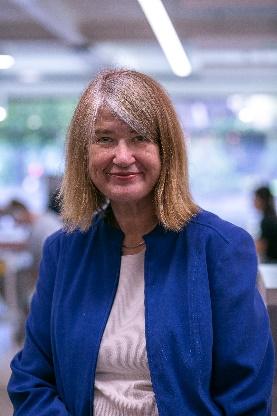
GESINE REINERT
Professor of Statistics, University of Oxford
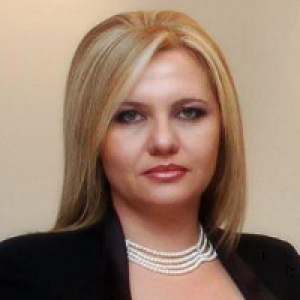
Aneta Antova Pesheva
General Manager in Ultra-Unet
BIOGRAPHY
Aneta Antova Pesheva has been the general manager of the group of IT companies “Ultra-Unet” for more than 35 years. Her company brought the Internet in North Macedonia for the first time 25 years ago and today it is also a system integrator with its own computer cloud system used by a large number of companies and institutions. She is currently the President of the strongest and most influential chamber of the ICT industry – MASIT and Honorary Consul of Canada in the Republic of North Macedonia. Throughout her career, Aneta has been involved in humanitarian organizations and is constantly striving to elevate women in the corporate world and society as well as to encourage as many young girls as possible to get involved and build careers in the STEM area.
ABSTRACT
As a profiled leader and business owner in the ICT industry for more than 35 years, Aneta will speak from her professional experience and the benefits and challenges of the industry. She will also address the mechanisms for gender equality she has implemented in her company and discuss how to encourage women to take more leadership positions in the industry. Supported by the most recent data and development plans, she will present the current ICT landscape in our country and our position in the region and globally. She will also address the new IT trends and AI tools that are inevitably coming, how they might affect the work force and human resources in the future, as well as the close relationship between digitalization and circular economy.
TOPIC: SYNTHETIC NETWORKS
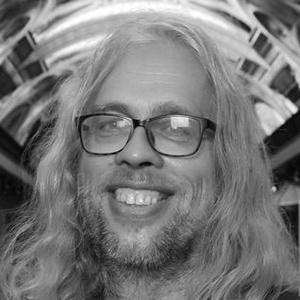
Thorsten Altenkirch
Professor for Computer Science, University of Nottingham
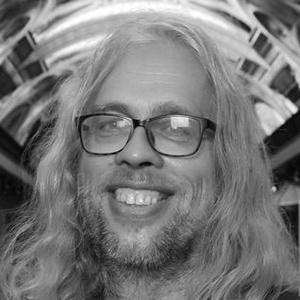
Thorsten Altenkirch
Professor for Computer Science at the University of Nottingham.
BIOGRAPHY
His research interest are in Type Theory and constructive logic and their application in proof assistants and programming languages. He has published a book on conceptual programming in Python (with Isaac Triguero) and is known for youtube videos on programming and other subjects in the Computerphile series.
TOPIC: Why dependent types matter
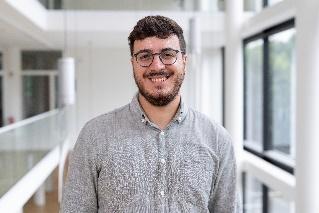
Aleksandar Bojchevski
Professor for Computer Science, University of Cologne
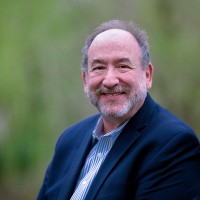
Paul Kayne
Vice President, Biological Sciences at Palatin Technologies
BIOGRAPHY
Dr. Paul Kayne has an extensive track record of innovation enabling pharmaceutical R&D and life-cycle management. Combining aspects of biology, engineering, imaging, and informatics provides a platform for this innovation. He is currently VP of Biological Sciences at Palatin, a company developing a new paradigm for treating inflammatory diseases. Prior to joining Palatin, Dr. Kayne held several roles at Bristol-Myers Squibb, most recently Head of Discovery Genomics & Proteomics. Previously, Dr. Kayne built one of the earliest microarray teams while at SmithKline Beecham and was a member of the Research Faculty at the California Institute of Technology. Dr. Kayne received his Ph.D. in molecular biology from the University of California, Los Angeles and his B.A. in molecular biology/biochemistry from the University of California, Santa Barbara.
TOPIC: Machine Learning with Guarantees
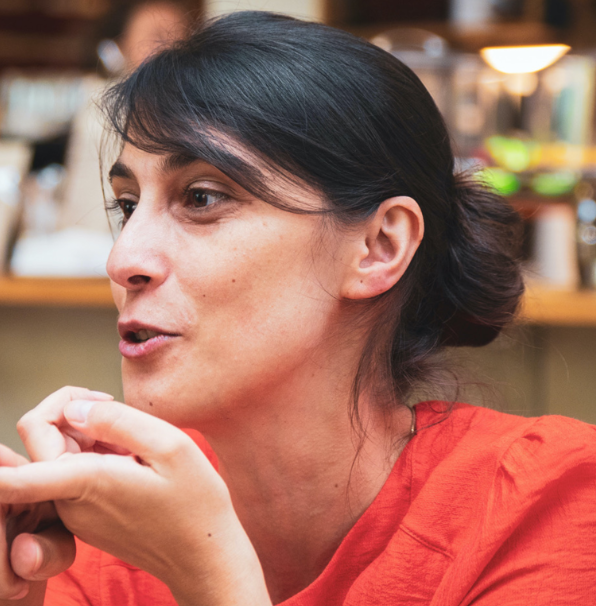
marija slavkovik
Professor for Computer Science, University of Bergen
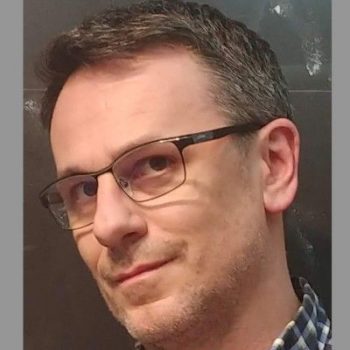
DEJAN ZVEKIC
Chief of Digitalization at Material Exchange
BIOGRAPHY
Dejan Zvekic is a prominent figure in the IT startup scene, known for his strategic vision and collaborative spirit. As Chief of Digitalization at Material Exchange, he is at the forefront of transforming the fashion industry through innovative digital sourcing solutions, effectively managing international teams to streamline production processes.
Dejan’s entrepreneurial journey is marked by significant achievements, including expanding Plugin76 from a small project to a company that was an object of successful acquisition by PTC Inc., a global company valued at over $10 billion.
He holds advanced degrees in marketing management, information systems, and an Executive MBA, blending strategic planning with market awareness.
DETAILS
The global pandemic has led to a ‘pivot’ to digital learning in many sectors of many countries, in schools, colleges and universities. My work with the UK Edtech Hub, British Council and Commonwealth of Learning suggests this response to the pandemic has been pedagogically conservative within those schools, colleges and universities, and furthermore may be increasing digital divides and educational disadvantage for those individuals, communities and cultures that are ignored, oppressed or poorly served by those schools, colleges and universities. My research explores in which innovative informal digital learning can help and support.
TOPIC: Why is AI a social problem in 2023?